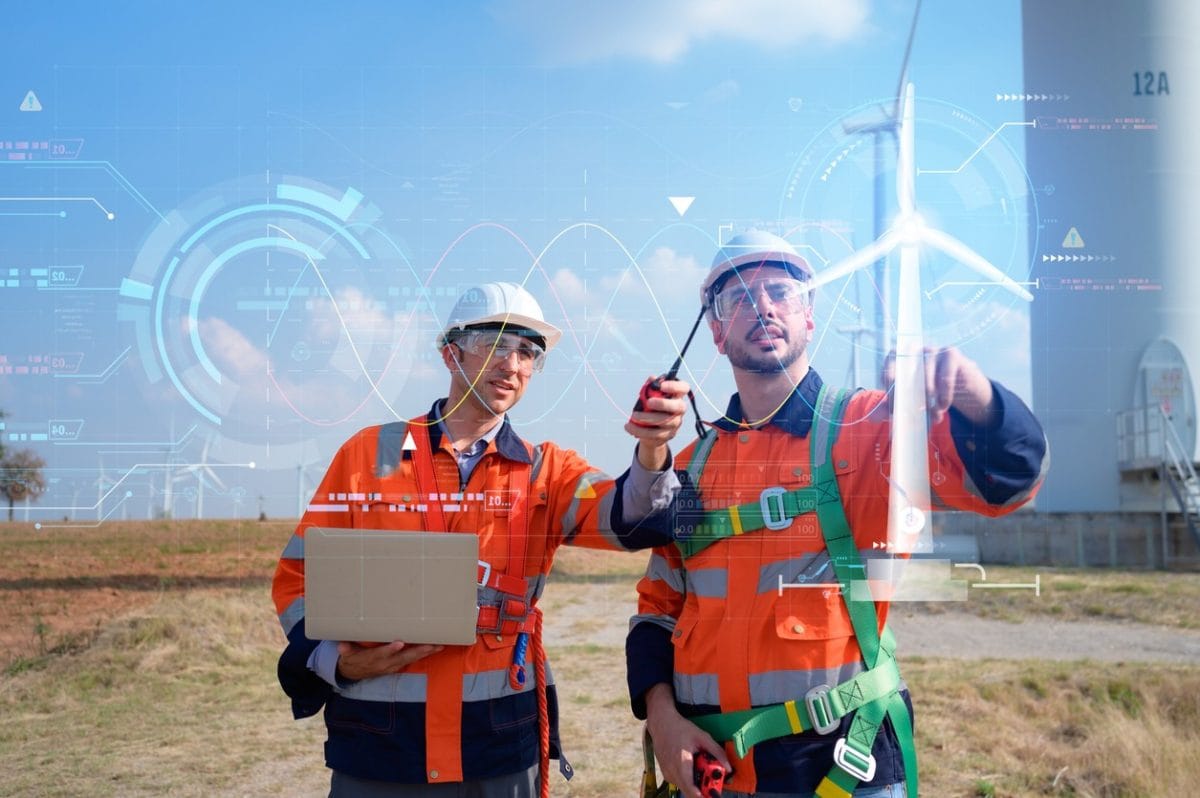
The future of AI in power and gas markets
AI: Reshaping power markets
The potential for AI to transform power and gas markets is hard to overstate.
As the world becomes increasingly electrified and power markets grow in complexity with the increasing penetration of renewables, AI could well revolutionise the electricity industry.
It is already showing real promise for enhancing power demand forecasts, optimising electricity flow, and more effectively balancing supply and demand. National energy companies are using AI for predictive grid maintenance and operational efficiencies, and AI is showing its potential value in energy trading. From weather forecasting to news analysis, it is becoming a powerful tool for making more efficient trading decisions, and that will only grow in importance.
Despite these advances, powering this revolution will still present significant challenges. Goldman Sachs predicts that power demand growth from data centres in the US will rise at a compound annual growth rate (CAGR) of 15% every year to 2030. AI data centres will go from a small part of overall power demand growth to a key driver almost overnight in relative terms.
The question is, how much energy will be needed to fuel this expected surge in demand? Will it be a moment for US natural gas to shine as an important enabler to sustain the AI boom? And how will AI reshape the whole power market landscape?
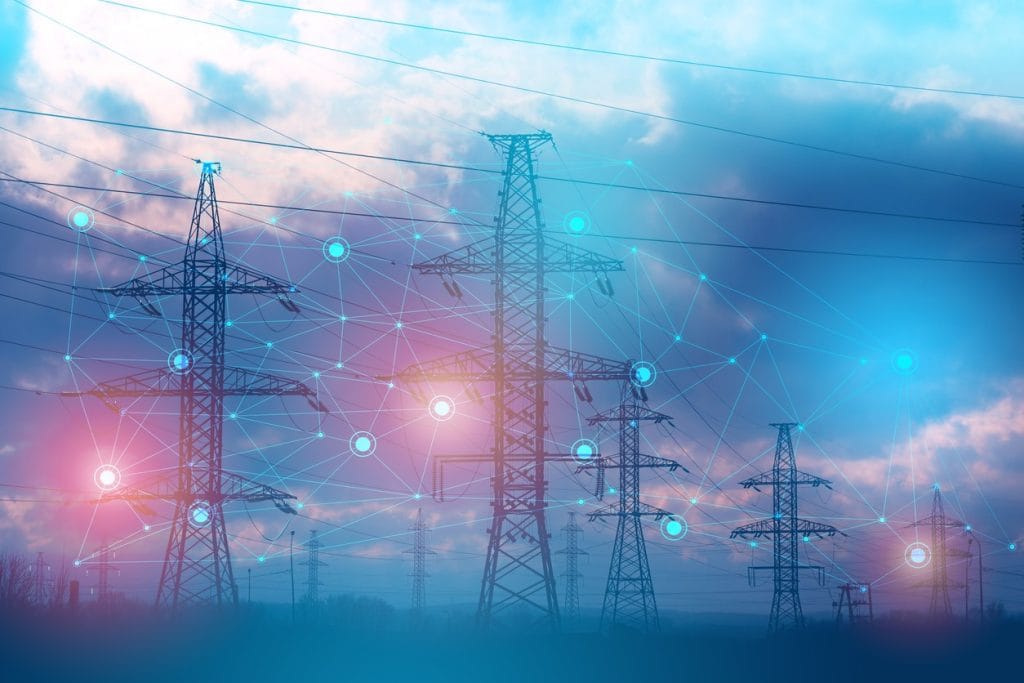
The growth in demand
Power demand growth in developed countries normally follows a fairly steady upward trajectory.
In the US, annual power generation over the last twenty years has averaged a growth rate of less than 0.5% growth. Total global energy consumption continues to grow, but it does seem to be slowing — averaging around 1% to 2% per year, compared to 4-6% in the decades post-World War II.
However, with the expansion of AI and the construction of new data centres, this steady growth pattern is poised for a dramatic shift. The world’s data centres are already significantly increasing their energy usage. In 2022, they used 460 terawatt hours (TWh) of electricity, but the International Energy Agency (IEA) expects this to double by 2026. By then, data centres could be using a total of 1000 TWh annually. As the IEA notes, this demand is roughly equivalent to Japan’s current electricity consumption. This is a huge wave of new energy demand that will push electricity grids to the limit.
“(AI’s energy needs won’t be met) without a breakthrough. We need fusion, or we need radically cheaper solar plus storage, or something, at massive scale – a scale that no one is really planning for.” – Sam Altman, CEO, Open AI
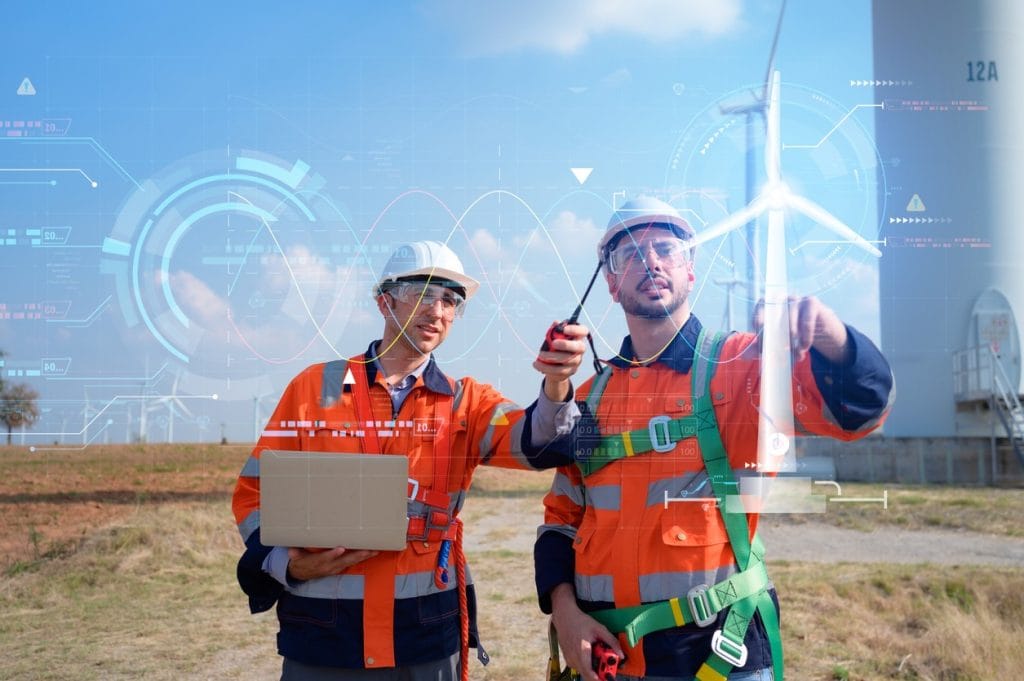
AI demand
AI models are energy-intensive to train and run.
Training complex models involves vast datasets and iterative computations, all of which demand significant power. Additionally, data centres housing AI systems require substantial energy for both computation and cooling.
According to the IEA, a single ChatGPT query requires 2.9 watt-hours of electricity, compared with 0.3 watt-hours for a Google search. This highlights the substantial energy requirements of AI technologies.
The energy demand required for AI is already accelerating, with an estimated annual growth rate between 26% and 36%. This rapid increase is driven by the expanding use of AI across multiple industries, the growing complexity of AI models, and the continuous demand for real-time data processing. As AI becomes more integral to everyday operations and innovations, addressing its escalating energy needs has become critical.
Developing more efficient algorithms that need less energy, optimising hardware performance, and investing in sustainable data centre infrastructure are essential to manage and mitigate the environmental and wider economic impact of AI’s rising energy consumption.
Just hoping that AI will solve some of these problems alone is not enough.
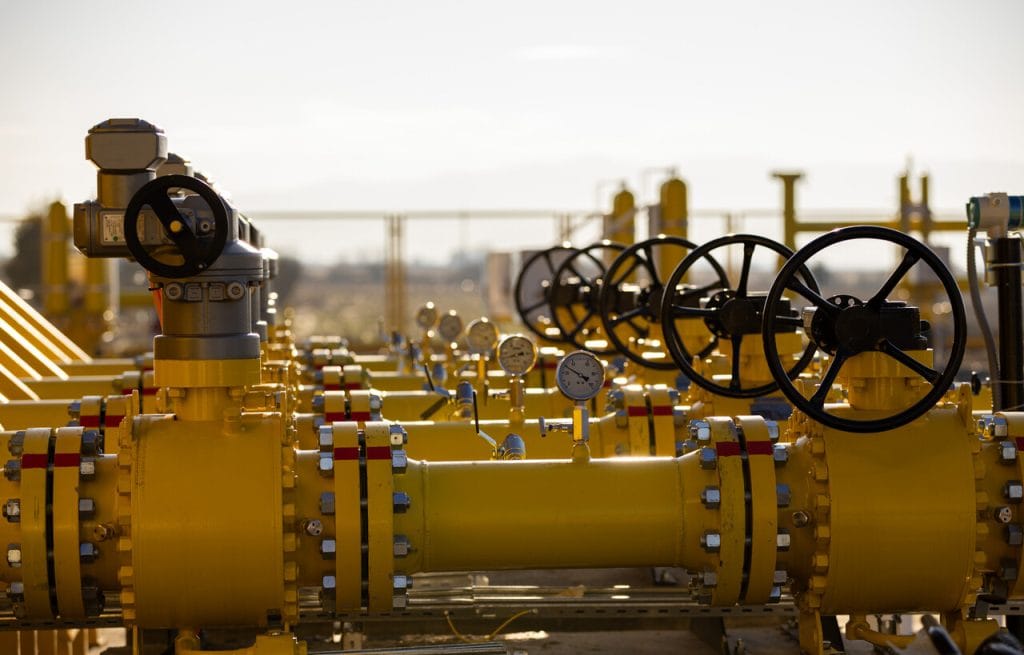
Increasing role for US Nat Gas
Large language models and AI technologies will continue to grow and need reliable, continuous, and sustainable power. Renewable energy sources, while essential for a sustainable future, face limitations due to their intermittent nature.
Without the new breakthrough that Sam Altman is looking for, natural gas may provide the near-term consistent baseload power required to support the growth of AI and data centre scaling.
Undoubtedly, gas will play a significant role in supporting AI’s future growth, how significant is still up for debate.
Demand growth forecasts in the US range from 3 billion cubic feet per day (BCF/D) to as much as 10 BCF/D by 2030. Even if the growth is somewhere in the middle of that range, it still underscores the importance of natural gas in an AI and data-powered future economy. This anticipated demand surge, coupled with new liquefied natural gas (LNG) export terminals in the US coming online, suggests we may be entering a new phase of tightness in global natural gas markets.
In the US, natural gas prices have been largely disconnected from global prices for years. The Henry Hub futures price for US natural gas has been at inflation-adjusted all-time lows this year and is currently trading at ~ $2.1 per million British thermal units (MMBtu). That compares with the Japan/Korea Marker Futures price, which gives a sense of the price for LNG cargoes in East Asia at ~$12/MMBtu and Dutch TTF last-day futures at ~ $10/MMBtu at the time of writing.
Bearish price action
Apart from a few notable periods, US gas prices have been largely suppressed in the two decades of fracking shale formations. Even compared to that low-price environment, this year has been particularly bearish.
An unusually warm winter and record output in 2023 has pushed natural gas prices to their lowest levels of the shale era. However, that may be about to change, and US natural gas may finally find more bullish momentum. This is supported by the acceleration of electricity demand from AI, data centres, and the electrification of industry in the long term, new LNG export capacity coming online this year and in 2025 and some indications that supply growth from the shale basins is slowing.
The hope is that these catalysts could help lift US natural gas prices out of the doldrums and unlock natural gas’s undoubted potential to play a crucial role in supporting AI and the clean energy transition for the rest of the decade.
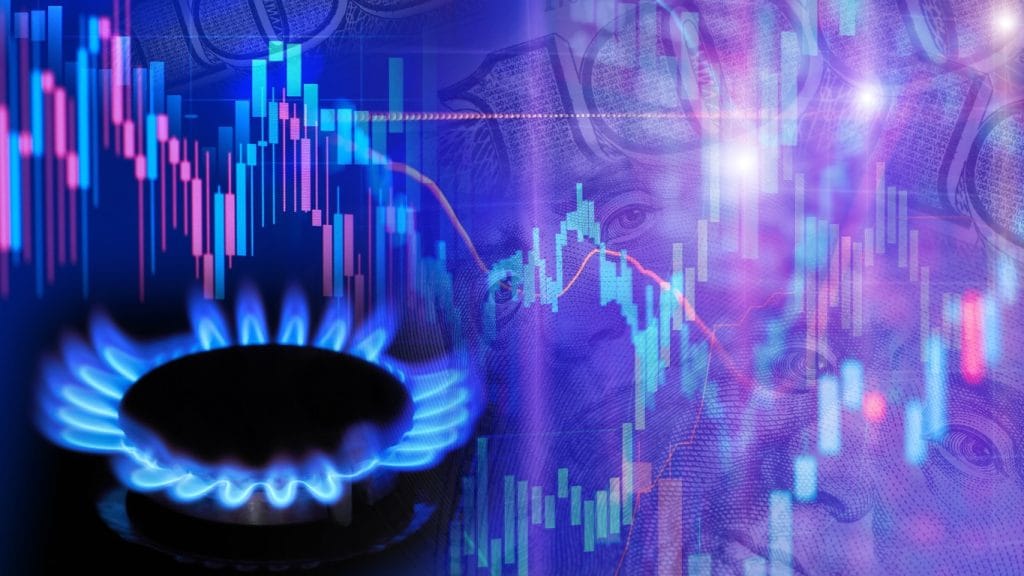
Creating more efficient power markets
AI will be one of the main drivers of the natural gas demand story, but the technology is already playing a key role in creating more efficient power markets. AI can enhance power demand forecasting by analysing vast amounts of data, including weather patterns, consumption trends, and economic indicators. This allows for better planning and reduces the need for excess capacity. Google’s AI research subsidiary, DeepMind, has used this to great effect to maximise the value of Google’s wind farms since 2019.
By training a neural network with publicly available weather forecasts and historical turbine data, the DeepMind system can forecast wind power output 36 hours before actual generation. These forecasts enable the model to recommend optimal hourly delivery commitments to the power grid a full day in advance. This increases the value of the output, as energy sources that can be scheduled to deliver specific amounts of electricity at predetermined times are more valuable to the grid.
DeepMind estimated in 2019 that its machine learning boosted the value of Google’s wind energy by roughly 20% compared to the baseline scenario of no time-based commitments to the grid. The French utility company Engie has now trialled the technology with its German wind portfolio.
AI also has the potential to improve grid management by optimising electricity flow, preventing outages, and more effectively integrating renewable energy sources, balancing supply and demand in real-time. Predictive maintenance is another critical application in which AI is already monitoring energy infrastructure to predict and prevent failures. Companies such as E.ON and Enel are using AI to predict maintenance needs, significantly reducing outages and operational costs.
DeepMind estimated in 2019 that its machine learning boosted the value of Google’s wind energy by roughly 20%
AI in trading power and gas
AI is also influencing power and gas trading, supporting traders to operate more effectively in the modern complex and fast-paced energy markets. AI models enable quick and accurate estimates of market variables by analysing historical data and relevant parameters like weather conditions.
These models predict trends in prices, demand, and supply volumes and identify competitors’ behaviour patterns. AI also helps simulate grid conditions to determine the need for interventions, optimising market positions for ancillary services.
However, it is important to note the limitations: historical data isn’t necessarily an accurate representation of the current or future grid, and obtaining useful data to train these models can be challenging.
For example, in our own testing with AI weather models, we’ve found that these AI models offer many benefits but with clear limitations, including the accuracy of extreme weather events or predicting more localised weather at a higher resolution. We don’t believe that AI will replace traditional numerical weather prediction (NWP) models any time soon, and the best forecasts will almost certainly come from AI and NWP working together. Still, AI weather modelling is already proving to be a hugely powerful tool for commodities trading, particularly in renewables.
AI’s ability to process and analyse written language also offers significant advantages in energy trading. By analysing news and reports, AI can anticipate market impacts from economic, financial, and political developments, allowing traders to make informed, strategic decisions.
AI weather modelling is already proving to be a hugely powerful tool for commodities trading, particularly in renewables
Realising AI’s potential
AI will drive tangible innovations in how we generate, manage, and trade power. However, to realise this potential, the industry must navigate the challenges of increased energy demand, leverage the benefits of natural gas, and continue to innovate towards more sustainable energy solutions. As AI models become more complex and their applications more widespread, the energy required to support these systems will grow exponentially, and there needs to be a transparent conversation about how we power that growth. AI is essential to create a more efficient, resilient and sustainable energy landscape of the future but renewables alone won’t be enough and natural gas and nuclear must play a key role to bridge the gap. They can support the expansion of AI and data centres while renewable technologies continue to develop and scale.
The synergy between AI and energy will be a cornerstone of the Fourth Industrial Revolution. By addressing the challenges of increased energy demand and integrating a mix of energy sources, the industry can ensure that AI plays a leading role in driving the transformation towards a more efficient and sustainable energy future.